Machine Learning and Misinformation
Varun Dashora | July 5, 2022
Artificial intelligence can revolutionize anything, including fake news.
Misinformation and disinformation campaigns are top societal concerns, with discussion about foreign interference through social media coming to the foreground in the 2016 United States presidential election [3]. Since a carefully crafted social media presence garners vast amounts of influence, it is important to understand how machine learning and artificial intelligence algorithms can be used in the future in not just elections, but also in other large-scale societal endeavors.
Misinformation: Today and Beyond
While today’s bots lack effectiveness in spinning narratives, the bots of tomorrow will certainly be more formidable. Take, for instance, Great Britain’s decision to leave the European Union. Strategies mostly involved obfuscation instead of narrative spinning, as noted by Samuel Woolley, a Professor of University of Texas-Austin who investigated Brexit bots during his time at the Oxford Internet Institute [2]. Woolley notes, “the vast majority of the accounts were very simple,” and functionality was largely limited to “boost likes and follows, [and] to spread links” [2]. Cutting-edge research indicates significant potential for fake news bots. A research team at OpenAI working on language models outlined news generation techniques. Output from these algorithms is not automatically fact-checked, leaving these models free reign to “spew out climate-denying news reports or scandalous exposés during an election” [4] With enough sophistication, bots linking to AI-generated fake news articles could alter public perception if not checked properly.
Giving Machines a Face
Machine learning has come a long way in rendering realistic images. Take, for instance, the two pictures below. Which one of those pictures looks fake?

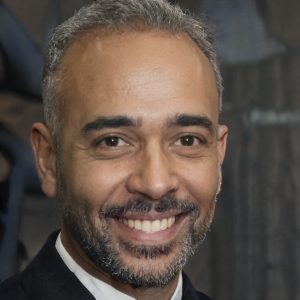
You might be surprised to find out that I’ve posed a trick question–they’re both generated by an AI accessible at thispersondoesnotexist.com [ 7]. The specific algorithm, called a generative adversarial network, or GAN, looks through a dataset, in this case of faces, in order to generate a new face image that could have feasibly been included in the original dataset. While such technology inspires wonder and awe, it also represents a new type of identity fabrication capable of contributing to future turmoil by giving social media bots a face and further legitimizing their fabricated stories [1]. These bots will show more sophistication than people think, which makes sifting real news from fake news that much more challenging. The primary dilemma posed questions and undermines “how modern societies think about evidence and trust” [1]. While bots rely on more than having a face to influence swaths of people online, any reasonable front of legitimacy helps their influence.
Ethical Violations
In order to articulate the specific ethical violations present, the Belmont Report is crucial to understand. According to the Belmont Report, a set of ethical guidelines used to evaluate the practices of scientific studies and business ventures, the following ideas can be used to gauge ethical harm: respect of individual agency, overall benefit to society, and fairness in benefit distribution [6]. The respect tenet is in jeopardy because of the lack of consent involved in viewing news put out by AI bots. In addition, the very content that these bots put out potentially distorts informed consent for other topics, creating ripple effects throughout society. The aforementioned Brexit case serves as an example; someone contemplating their vote on the day of the referendum vote would have sifted through a barrage of bots retweeting partisan narratives [2]. In such a situation, it is entirely possible that this hypothetical person would have ended up being influenced by one of these bot-retweeted links. Given the future direction of artificially intelligent misinformation bots, fake accounts and real accounts will be more difficult to distinguish, giving rise to a more significant part of the population being influenced by these technologies.
In addition, the beneficence and fairness clauses of the Belmont report are also in jeopardy. One of the major effects of AI-produced vitriol is more polarization. According to Philip Howard and Bence Kollanyi, social media bot researchers, one effect of increased online polarization is “a rise in what social scientists call ‘selective affinity,’” which means people will start to shut out opposing voices due to the increase in vitriol [3]. These effects constitute an obvious violation of beneficence to the broader society. In addition, it is entirely possible that automated narratives spread by social media bots target a certain set of individuals. For example, the Russian government extensively targeted African Americans during the 2016 election [5]. The differential in impact means groups of people are targeted and misled unfairly. With the many ethical ramifications bots can have on society, it is important to consider mitigations for artificially intelligent online misinformation bots.
References
– [1] https://www.theverge.com/tldr/2019/2/15/18226005/ai-generated-fake-people-portraits-thispersondoesnotexist-stylegan
– [2] https://www.technologyreview.com/2020/01/08/130983/were-fighting-fake-news-ai-bots-by-using-more-ai-thats-a-mistake/
– [3] https://www.nytimes.com/2016/11/18/technology/automated-pro-trump-bots-overwhelmed-pro-clinton-messages-researchers-say.html
– [4] https://www.technologyreview.com/2019/02/14/137426/an-ai-tool-auto-generates-fake-news-bogus-tweets-and-plenty-of-gibberish/
– [5] https://www.bbc.com/news/technology-49987657
– [6] https://www.hhs.gov/ohrp/regulations-and-policy/belmont-report/read-the-belmont-report/index.html
– [7] thispersondoesnotexist.com