The Credit Conundrum
Anonymous | October 14, 2022
A person’s credit score is an important personal data feature that lenders use to evaluate a borrower’s ability to pay back a loan (i.e. creditworthiness). The unfortunate reality is that most consumers don’t have a grasp on the nuances of the credit score model, the most prominent of which was developed by Fair Isaac Corp. (FICO). Credit scores can determine both an individual’s ability to get a loan (e.g. auto loan, mortgage, business loan, student loan, etc.) the interest rate associated with that loan, and the amount of deposit required for larger purchases. FICO has categorized estimated creditworthiness with the following ranges: Excellent: 800–850, Very Good: 740–799, Good: 670–739, Fair: 580–669, Poor: 300–579.
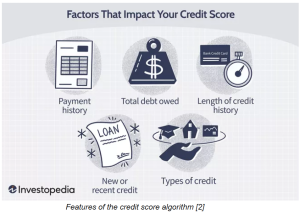
Rarely does the average consumer comprehend the factors that affect their FICO credit score, and it’s quite possible that many consumers don’t know their FICO score or how to check for it. The reality is, a credit score below 640 is usually considered “subprime” and puts the borrower in a dangerous position of falling into a debt trap. “Data shows that more than 1 in 5 Black consumers and 1 in 9 Hispanic consumers have FICO scores below 620; meanwhile, 1 out of every 19 white people are in the sub-620 category” [1]. Subprime borrowers frequently become the target of predatory lending which only exacerbates the unfortunate situation. A Forbes article, written by Natalie Campisi, asserts that the current credit scoring model has been negatively influenced by a long history of discriminatory practices. Algorithmic bias in the credit industry was acknowledged in 1974 when the “Equal Credit Opportunity Act disallowed credit-score systems from using information like sex, race, marital status, national origin and religion” [1]. However, the new credit score model evaluation criteria doesn’t take into account generations of socioeconomic inequity. Federal legislation has been passed in addition to the Equal Credit Opportunity Act to make the credit industry more transparent and equitable.
Despite these efforts on a federal level, the issue of algorithmic bias remains when credit agencies aggregate data points into individual credit scores. Generational wealth has passed disproportionately to white people, so the concept of creditworthiness should be reimagined with feature engineering for equity and inclusion. For example, “FinReg Labs, a nonprofit data testing center, analyzed cash-flow underwriting and the results showed that head-to-head it was more predictive than traditional FICO scoring. Their analysis also showed that using both the FICO score and cash-flow underwriting together offered the most accurate predictive model” [1].
Enhancing the fairness of the credit industry could prove pivotal to the advancement of disenfranchised communities. Credit scoring models ignore rental payment history, but they take housing payments into account when generating credit scores. This prevents many otherwise credit worthy individuals from improving their credit score due to the massive gap in homeownership between whites (74.5% by end of 2020) and non-white communities (44% by end of 2020) [1]. The FICO credit scoring model has gone through many iterations and a variant is used in about 90% lending cases [2]. However, lenders may use different versions of the algorithm to determine loan amounts, interest rates, payback period, and any deposits. Therefore, there’s a need for uniformity of credit standards across different lending opportunities to prevent lending bias. A recent Pew Research paper found that in New York City, over half of debt claims judgments/lawsuits affected individuals in predominantly black or hispanic communities, and 95% of the lawsuits affected people in low- to moderate-income neighborhoods [1]. “Using data that reflects bias perpetuates the bias, critics say. A recent report by CitiGroup states that the racial gap between white and Black borrowers has cost the economy some $16 trillion over the past two decades. The report offers some striking statistics:
● Eliminating disparities between Black and white consumers could have added $2.7 trillion in income or +0.2% to GDP per year.
● Expanding housing credit availability to Black borrowers would have expanded Black homeownership by an additional 770,000 homeowners, increasing home sales by $218 billion.
● Giving Black entrepreneurs access to fair and equitable access to business loans could have added $13 trillion in business revenue and potentially created 6.1 million jobs per year.” [1]
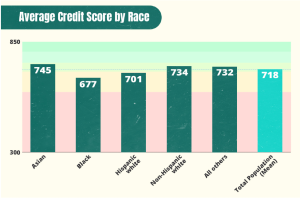
I’ve worked as a financial consultant for both Merrill Lynch Wealth Management and UBS Private Wealth Managment, so I have first-hand insight into the credit conundrum. The credit industry could be enhanced through the development of structured lending products. Furthermore, the bankers who develop these lending products should form criteria that accounts for both years of economic inequality and the reinterpretation of “creditworthiness”. Also, institutional banks should do financial literacy and credit workshops in disenfranchised communities and publish relevant content to remedy the credit disparity. Clients who employ financial consulting services are educated on how to leverage the banking system to reach their financial goals, but the vast majority of the U.S. population doesn’t qualify for personalized financial services. However, these same financial services organizations interface with the masses. Banks should cater to the masses to empower rather than to exploit the proletariat through discriminatory or predatory lending practices.
References
[1] From Inherent Racial Bias to Incorrect Data—The Problems With Current Credit Scoring Models – Forbes Advisor
[2] Credit Score: Definition, Factors, and Improving It
[3] What are the Texas Fair Lending Acts?
[4] Credit History Definition
[5] Subprime Borrower Definition
[6] Average Credit Score by Race: Statistics and Trends